Fastenerd (2022)
Machine Screw Identifier
MIT Product Design & Development Studio
with Ben Thomson, Bruce Crawford, Darron Sandifer, Hana Khalil,
Jacob Hopkins, Marwa AlAlawi, Ne Myo Han, Kevin Tang
The differences between imperial and metric machine bolts are slight, it is difficult and time consuming to identify and sort them by hand resulting in a lot of waste as most miscellaneous screws are thrown out in a professional shop environment. This project seeks to mitigate these issues.
Design Process
The design of the fastener identifier was based on the workflow of our users. Sketch models were created to determine the most appropriate workflow. The device was designed to speed up the user as much as possible, stay out of their way, and be as versatile as possible. The faster the user identified their fastener, the faster they can store it. Thus, speed and repeatability were the guiding principles behind design decisions. The detailed design considered the full user experience, from finding fasteners, to identifying them, and finally to storing them. The touch-points where users interact with the device were carefully placed to allow the users to effortlessly identify fasteners over and over again. We used the Agile methodology to find opportunities for systemic innovations and to keep us on track.
Pre-Alpha Prototype, featuring a functional camera and raspberry pi system
Sprint 1+2 Highlights
Testing out optical solutions (machine learning) and interfacing: The first direction that we decided to pursue is optical sorting. We were thinking about using a camera running on a machine learning model to identify and separate our fasteners.
This method is commonly used for industrial-scale part sorting with sophisticated alignment and image-capture systems on a conveyor belt setup. However, those machines would be too bulky and expensive for hobbyists and small shops. Therefore, we envision building a product that is compact and cheap, but has a robust algorithm to sort fasteners at a sufficient speed and accuracy. The machine learning model may be able to allow individual users to customize the sorter to specific projects and use cases.
Sprint 3+4 Highlights
Focusing on Hopper System and Form Factor
In the first half of the product development process, we were focused on a system that could potentially address a larger number of customer needs associated with fastener use but required significant added complexity. We were attempting to develop a fastener sorting tool that had the ability to singulate a fastener from a pile, present it to the optical measuring system for identification, and then sort it into a bin accordingly.
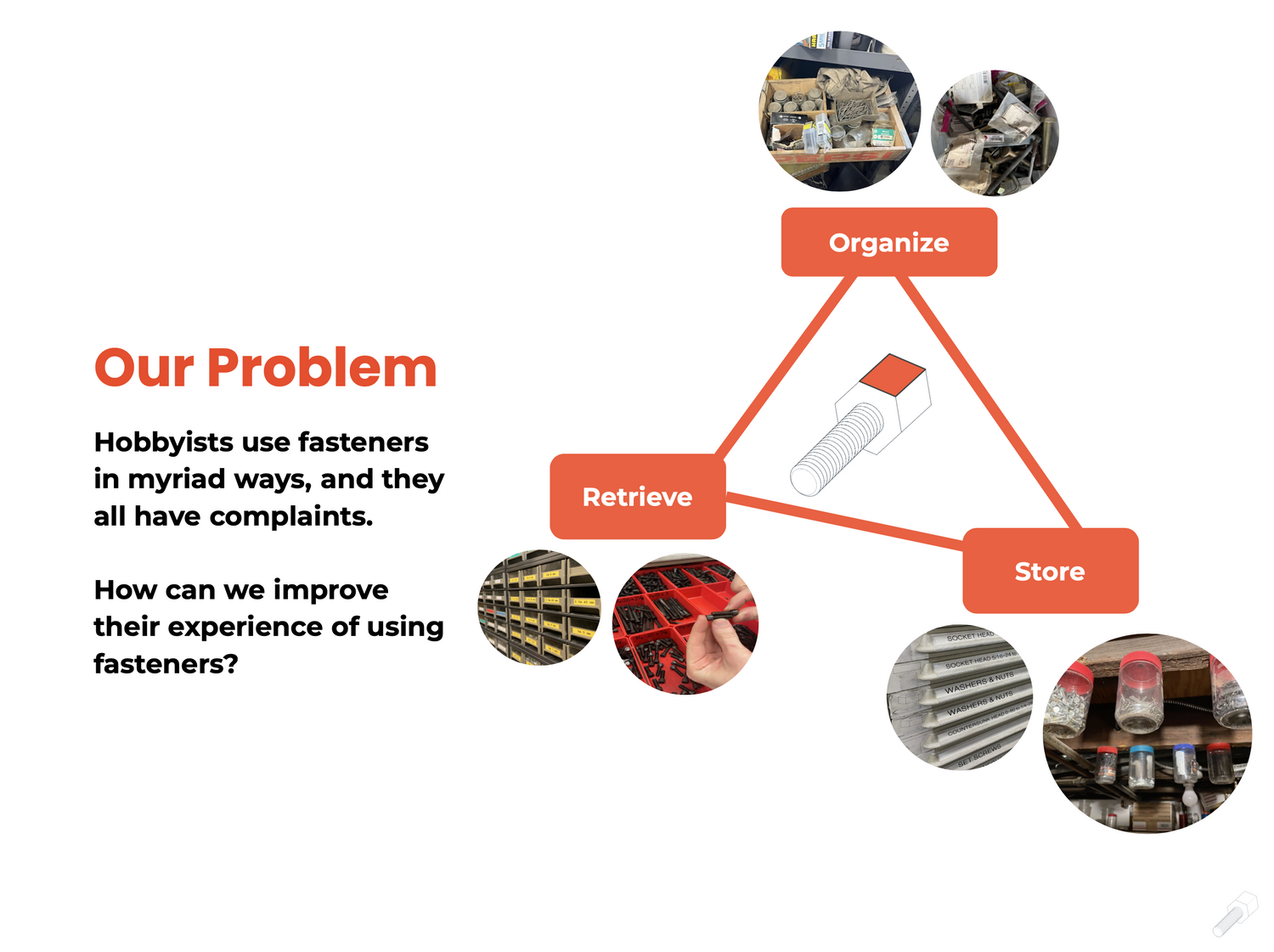


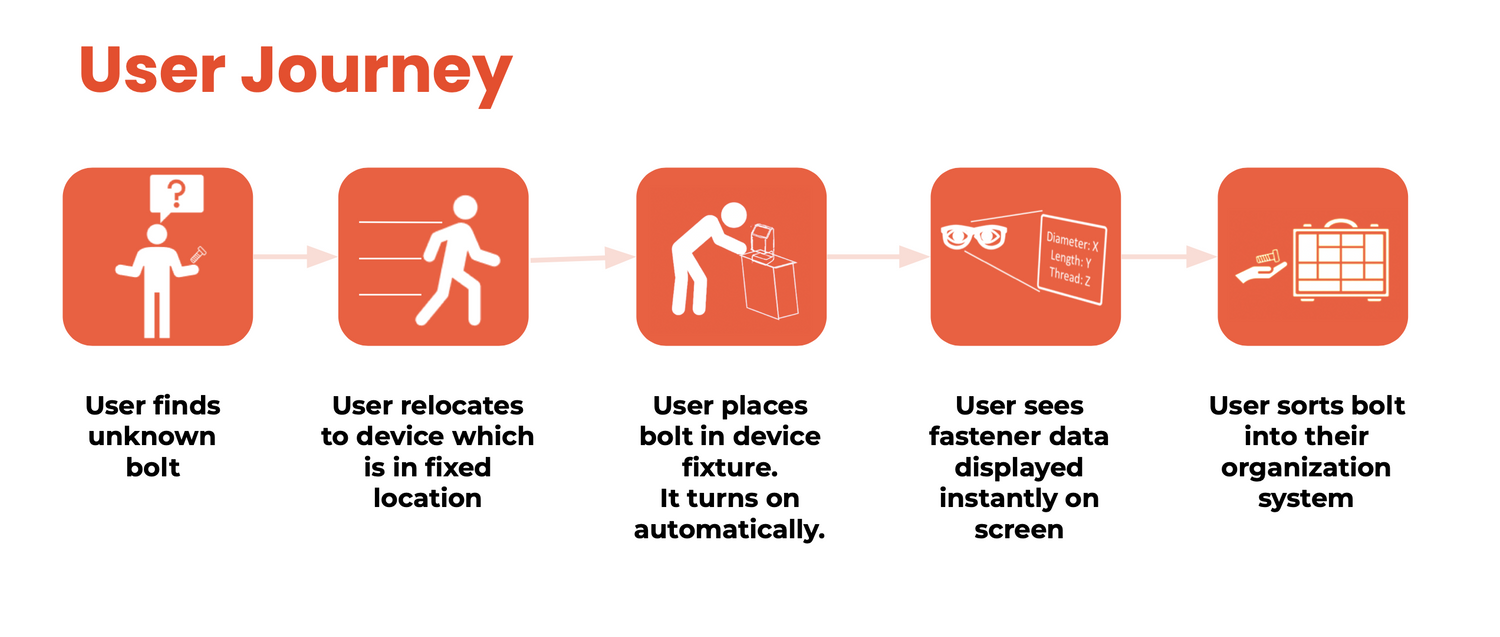
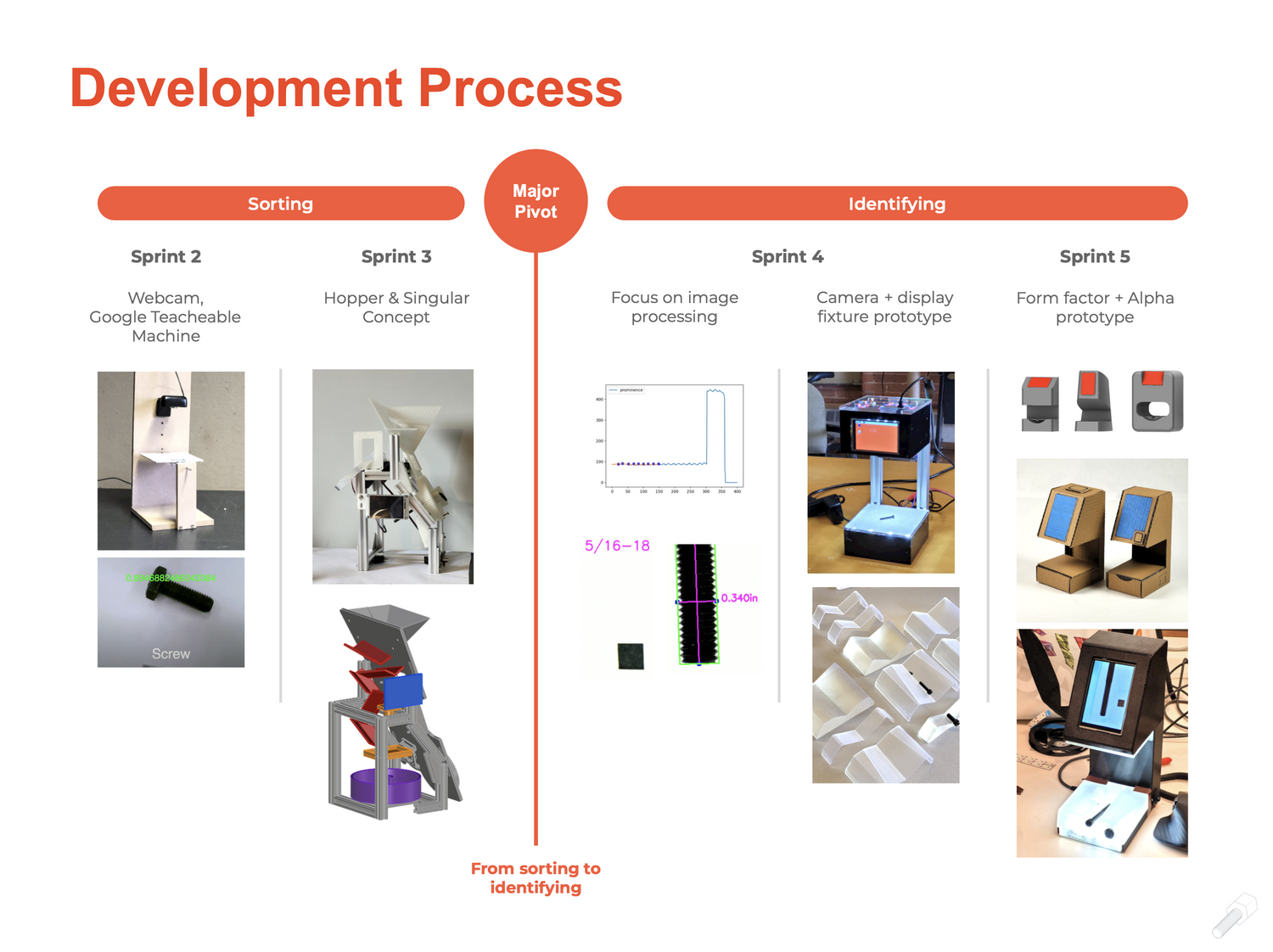
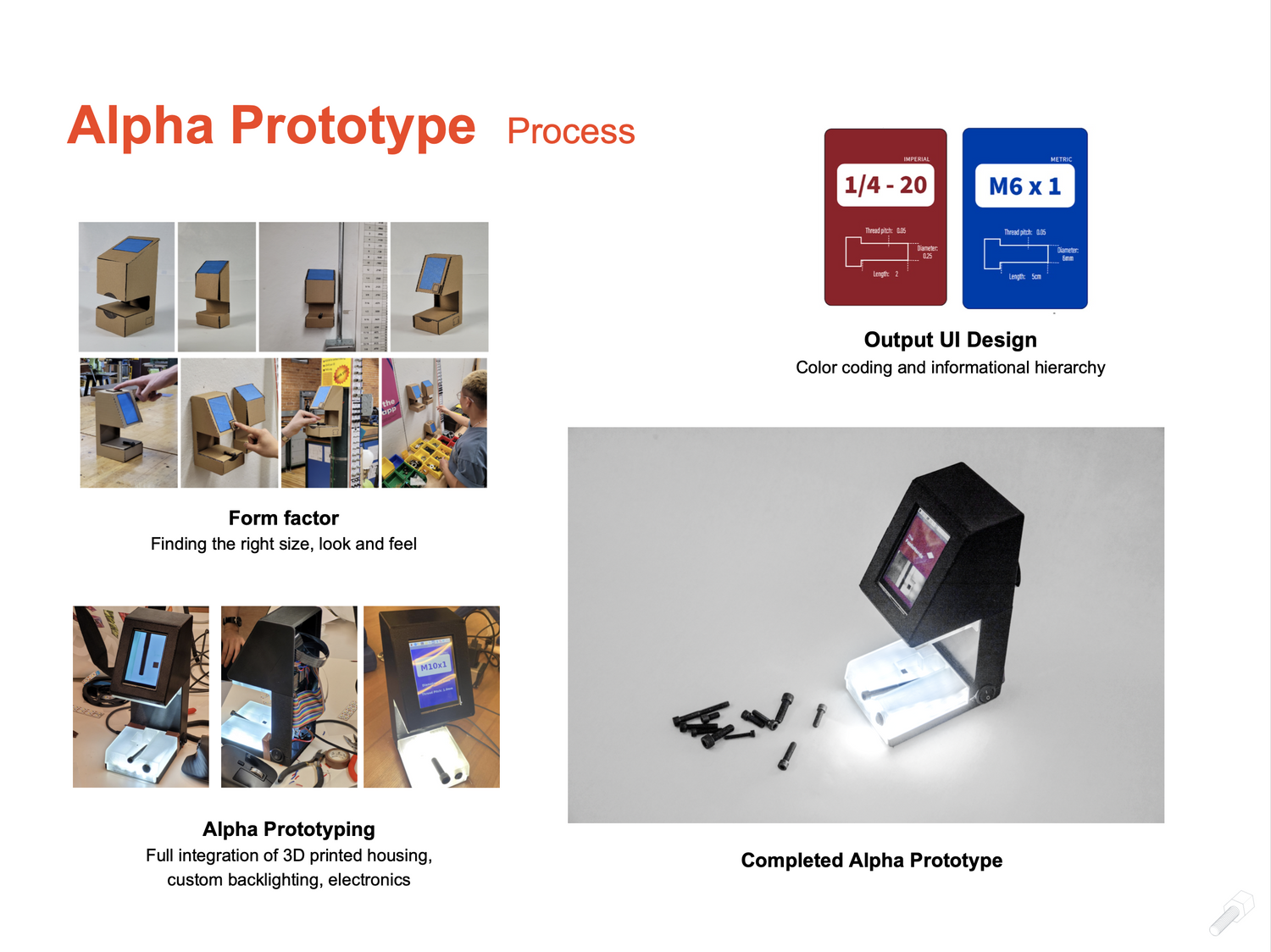
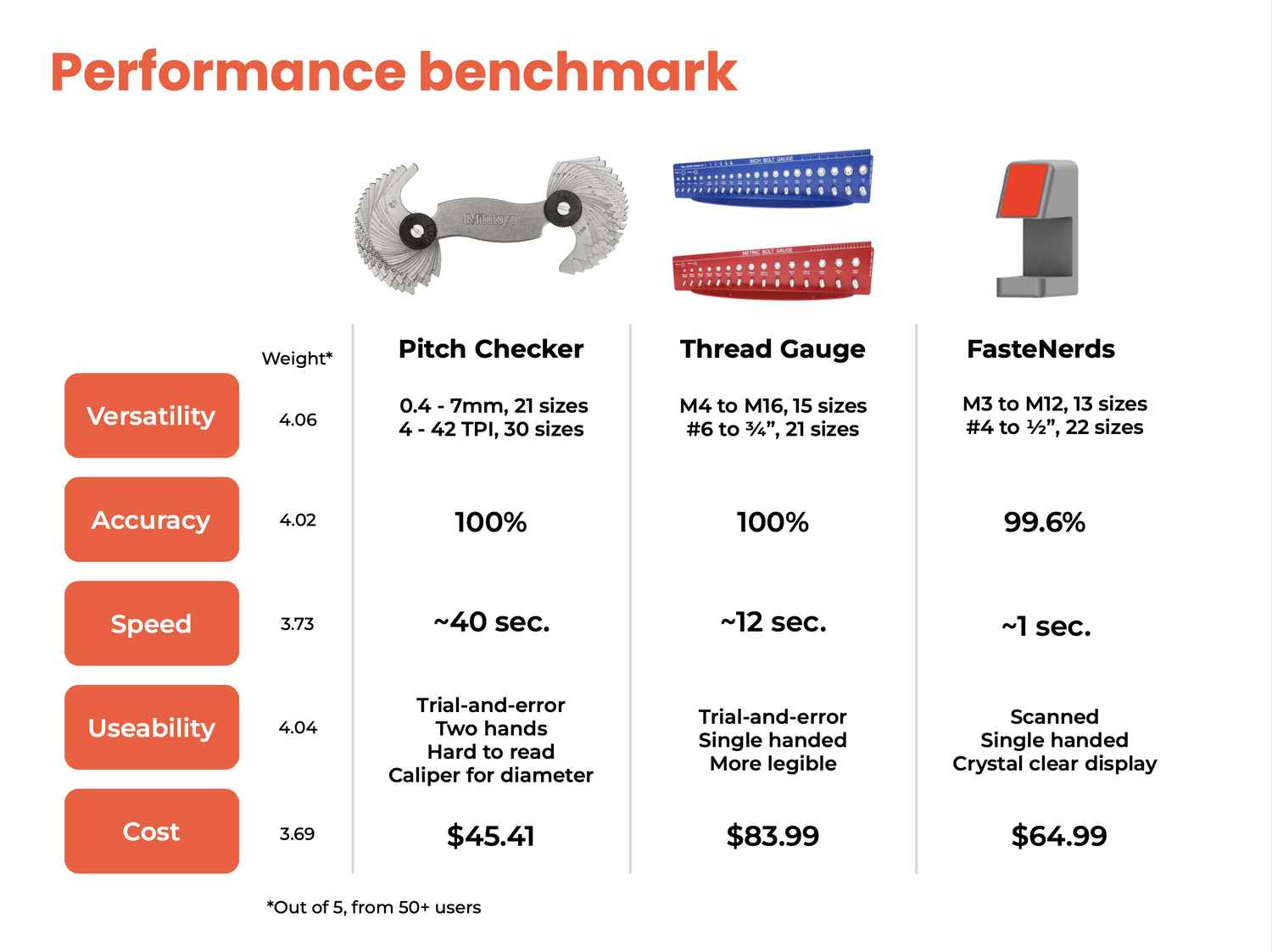

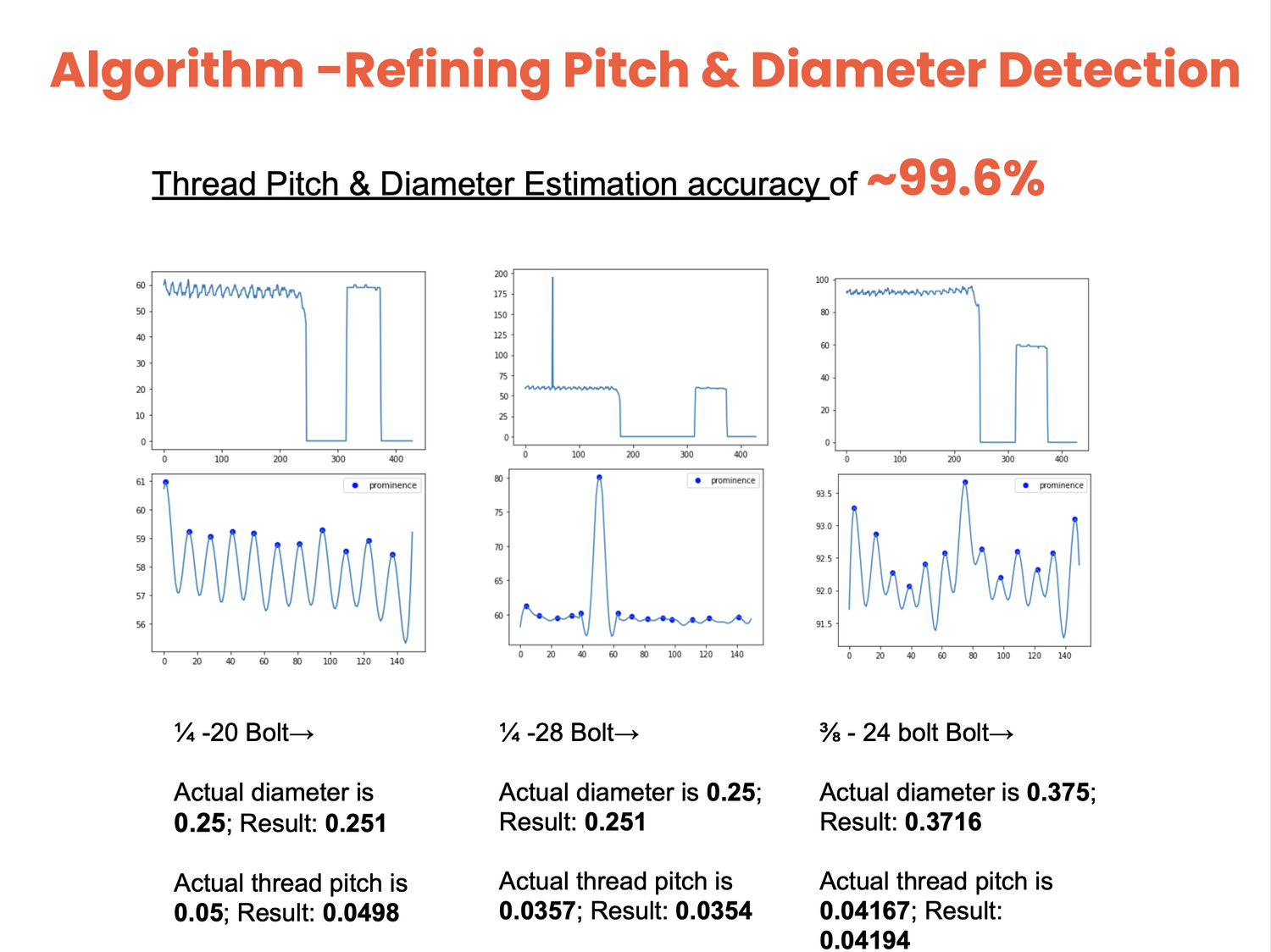

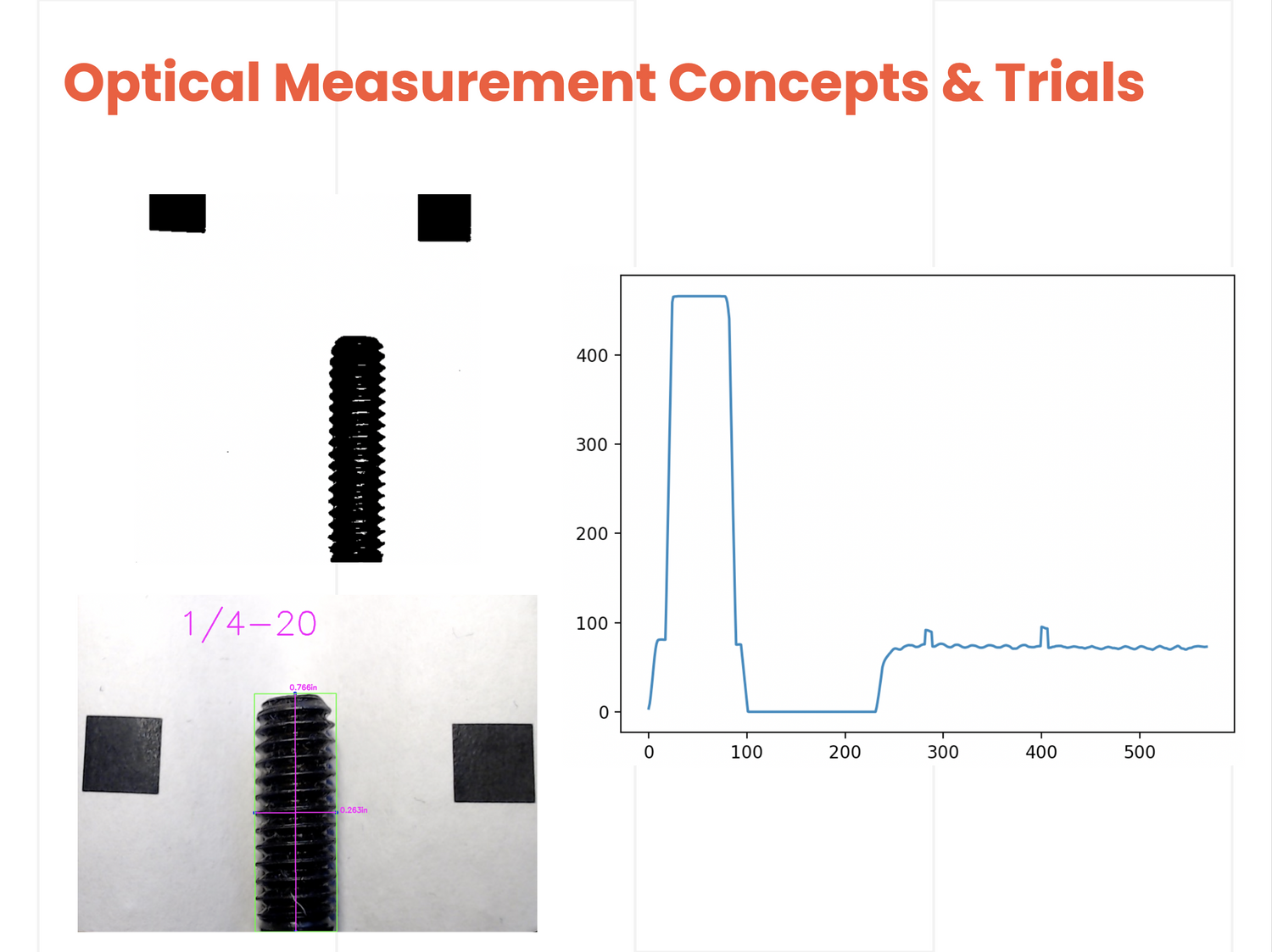
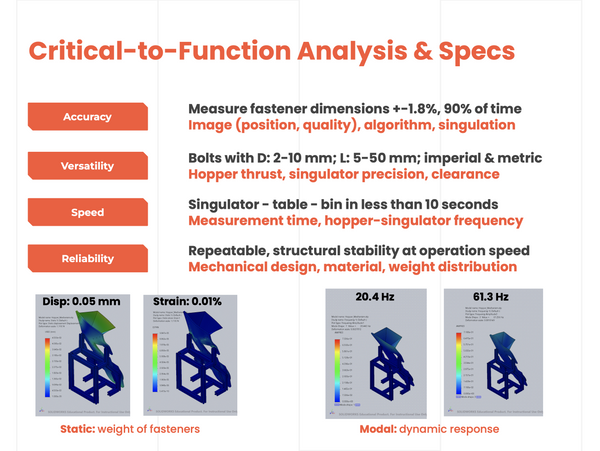
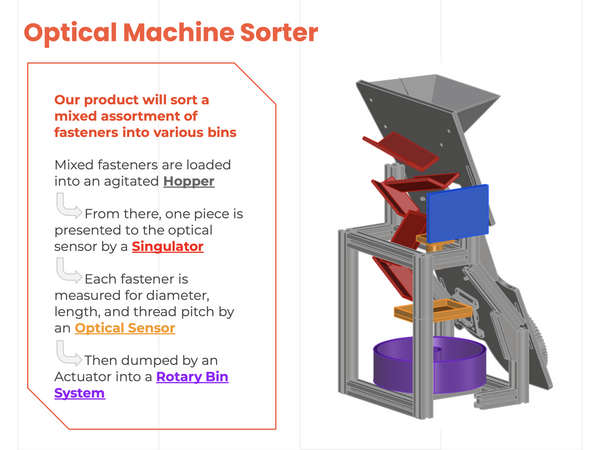
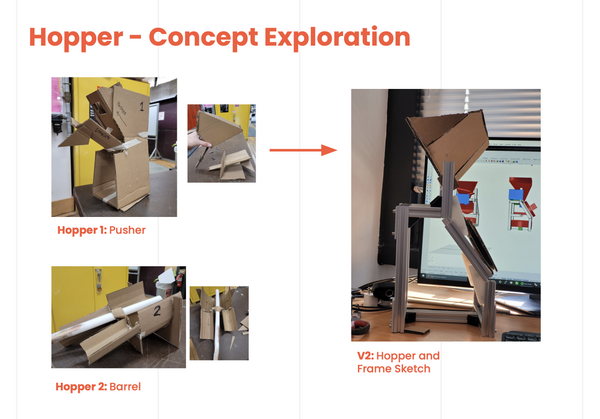